In the rapidly evolving world of imaging technology, camera type identification plays a crucial role in enhancing cameras’ performance and efficiency across various industries. One of the most promising methodologies in this area is VL2L (Variable Length to Length), a technique that automates determining camera types with precision. As camera technology advances, the VL2L determine camera type approach is set to redefine how we identify and classify cameras, impacting everything from consumer electronics to high-tech imaging in fields like medicine and security.
In this article, we will explore how VL2L works, why it is important, and how it is shaping the future of camera-type identification.
What is vl2l determine camera type?
VL2L, or Variable Length to Length, is a sophisticated analytical method used in data processing, particularly for identifying complex patterns. When applied to camera type identification, VL2L converts variable data from camera sensors into fixed-length representations that can be more easily analyzed. This process simplifies the often-complicated task of determining key sensor attributes, such as resolution, noise levels, and dynamic range, which are essential for identifying the type of camera in use.
By automating the process of camera type identification, VL2L reduces human error and speeds up decision-making. This is particularly valuable in industries where precise sensor selection is crucial, such as in the development of smartphones, medical imaging devices, and surveillance cameras.
Key Features of VL2L in Camera Identification
- Automated Data Processing: VL2L eliminates the need for manual camera-type identification by automating the analysis of sensor data.
- Pattern Recognition: The method recognizes specific patterns in the sensor data to accurately determine the type of camera sensor, whether it’s CCD or CMOS.
- Speed and Accuracy: VL2L speeds up the identification process and ensures high accuracy, reducing the risk of errors that can arise in manual inspection.
- Versatility: This method can be applied to a variety of camera types, from high-end professional cameras to consumer-grade smartphones.
How VL2L Determines Camera Type
The process of VL2L determining camera type involves several key steps:
- Data Collection: The first step is gathering data from the camera sensor, which includes key parameters such as pixel resolution, noise characteristics, light sensitivity, and spectral response.
- Signal Processing: VL2L algorithms are applied to convert the raw data into fixed-length forms. This step transforms the sensor information into easily comparable data points that highlight the unique features and characteristics of the camera sensor.
- Pattern Recognition: The fixed-length representations are then analyzed to identify specific patterns or signatures that correspond to known camera sensor types. This analysis is based on pre-existing data from known camera types, which allows for accurate sensor identification.
- Matching and Identification: Finally, the identified patterns are matched against a database of known camera sensor types, enabling the system to accurately determine whether the sensor is a CCD, CMOS, or another variant.
By following these steps, the VL2L determine camera type method provides a reliable and efficient way to identify camera sensors with minimal human intervention.
Advantages of Using VL2L for Camera Type Identification
1. Enhanced Precision and Accuracy
One of the most significant advantages of using VL2L to determine camera type is the enhanced accuracy it offers. Moreover, the technique’s reliance on pattern recognition and fixed-length analysis allows for precise identification of camera sensors, minimizing the chances of error. This is particularly useful in fields like medical imaging, where sensor accuracy can be the difference between a correct diagnosis and a misdiagnosis.
2. Reduced Costs
By automating the camera identification process, VL2L reduces the need for extensive human labor, cutting down on operational costs. This is a major benefit for industries that require high-volume camera production and testing, such as smartphone manufacturing.
3. Improved Speed
Speed is another crucial factor in the VL2L determining camera type approach. With traditional methods, identifying the type of camera sensor could take a considerable amount of time, particularly when dealing with large datasets. VL2L dramatically accelerates this process, allowing manufacturers to identify and classify camera sensors in real-time, boosting efficiency.
4. Scalability Across Industries
VL2L is highly scalable and can be adapted for various industries, from consumer electronics to automotive imaging. This flexibility ensures that the methodology remains relevant across a range of applications, driving innovation in fields that depend on accurate camera technology.
Challenges in Sensor Assurance Utilizing VL2L
As camera technology advances, the importance of sensor assurance—ensuring the quality and reliability of camera sensors—has never been greater. The VL2L determine camera type method has emerged as a promising solution for identifying and classifying camera sensors with high precision and speed. However, despite its advantages, the application of VL2L in sensor assurance comes with its own set of challenges.
In this article, we will explore the six key challenges in sensor assurance when utilizing VL2L technology and examine how these challenges can be addressed to improve sensor quality and reliability across various industries.
Complexity of Data Processing
Challenge Overview
The first challenge in sensor assurance using VL2L is the complexity involved in processing vast amounts of data. Camera sensors generate an enormous volume of data, including pixel-level details, light sensitivity, noise characteristics, and color accuracy. Additionally, transforming this raw data into meaningful patterns and identifying sensor types requires advanced signal processing techniques and considerable computational resources.
Why It’s a Challenge
While VL2L converts variable-length data into fixed-length representations for easier analysis, handling such large datasets in real-time can still be resource-intensive. The complexity increases when dealing with sensors designed for specialized applications, such as ultra-high-resolution sensors or those used in autonomous vehicles, where even the slightest discrepancy in data processing can lead to inaccuracies in sensor assurance.
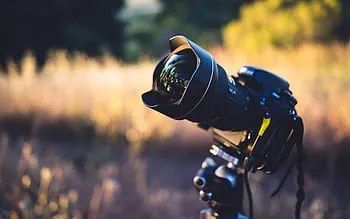
Sensor Degradation Over Time
Challenge Overview
Sensor degradation is an inevitable challenge in camera technology. Over time, camera sensors can deteriorate due to factors like exposure to extreme temperatures, humidity, or frequent use. The challenge for VL2L is to identify these degradations early and ensure that the sensor still meets the required performance standards.
Why It’s a Challenge
As sensors degrade, the data they generate may become inconsistent or unreliable. This poses a problem for VL2L-based sensor assurance, as the system may not always be able to detect subtle changes in sensor quality, leading to inaccurate identification and assessment of sensor performance.
Possible Solutions
- Continuous Monitoring: Implementing continuous monitoring systems that regularly check sensor performance can help identify signs of degradation before they become critical.
- Degradation Prediction Models: Using machine learning models to predict sensor degradation based on historical data can help prevent performance issues and improve long-term sensor assurance.
Applications of vl2l Determine Camera Type Identification
1. Smartphones and Consumer Electronics
One of the primary applications of VL2L in camera type identification is within the smartphone industry. As camera technology continues to be a major selling point for smartphones, manufacturers need to ensure that their devices feature high-performance sensors. The VL2L determine camera type method allows smartphone manufacturers to quickly and accurately identify and select the best sensors for their devices, improving image quality and overall user experience.
2. Medical Imaging
In the medical field, imaging devices such as MRI scanners, X-ray machines, and ultrasound systems rely on precise sensors to capture high-quality images. The VL2L method is crucial in this industry, ensuring that the correct sensor types are selected for each imaging device. This not only improves the accuracy of medical diagnoses but also enhances the reliability of medical equipment.
3. Surveillance and Security
In surveillance systems, especially in environments with varying lighting conditions, choosing the right camera sensor is essential. The VL2L determines camera type approach helps in selecting sensors that can perform well in different conditions, ensuring clarity and responsiveness in security cameras.
4. Automotive and Autonomous Vehicles
As the automotive industry shifts towards autonomous driving, camera sensors play a pivotal role in capturing real-time data for navigation and safety features. Additionally, the VL2L method allows car manufacturers to determine the most suitable camera sensors for their advanced driver assistance systems (ADAS), ensuring real-time data capture and enhanced vehicle safety.
The Future of Camera Type Identification with VL2L
The future of camera-type identification is set to be heavily influenced by advancements in VL2L technology. Additionally, as cameras continue to evolve, with higher resolutions and more sophisticated sensors, VL2L will need to adapt to keep pace with these advancements. Here are some emerging trends and expectations for the future:
1. Integration with AI and Machine Learning
The next step for vl2l to determine camera type technology is its integration with artificial intelligence (AI) and machine learning algorithms. By combining these technologies, VL2L can become even more accurate and efficient in identifying camera types. Additionally, machine learning models can be trained on vast datasets of camera sensors, allowing the system to continuously improve its identification capabilities.
2. Real-Time Data Processing
As computational power continues to increase, VL2L will be able to process data in real-time. Additionally, this means that camera-type identification can occur instantly, allowing for even faster decision-making in industries like automotive and surveillance, where real-time analysis is critical.
3. Advancements in Camera Sensor Technology
As camera sensors evolve, with new technologies like quantum dot sensors and organic image sensors on the horizon, the VL2L method will need to evolve as well. These new types of sensors will require more complex identification processes, and VL2L will play a key role in adapting to these changes.
4. Expanded Applications in Emerging Industries
Beyond traditional industries, VL2L is expected to find applications in emerging fields like space exploration and augmented reality. Additionally, in these industries, accurate and efficient camera-type identification will be critical for capturing high-quality images in challenging environments.
Conclusion
The vl2l determine camera type (Variable Length to Length) method is set to transform camera type identification across various industries. Moreover, by automating sensor data analysis, VL2L enhances accuracy, reduces costs, and speeds up processing, benefiting applications in smartphones, medical imaging, surveillance, and autonomous vehicles. Its precision minimizes errors and supports better decision-making in high-performance imaging.
Despite its advantages, challenges like data processing complexity and sensor degradation need addressing for sustained reliability. Additionally, future advancements will likely include integrating VL2L with AI and machine learning, enhancing real-time processing, and adapting to new sensor technologies. The scalability and versatility of VL2L position it as a key player in the future of imaging systems.